Real-Time Incident Detection Using Social Media Data
Team: Sean Qian (PI, CMU), Yiming Gu (CMU)
Funding source: PennDOT, T-SET
Start/End time: 2014-2015
​
The effectiveness of traditional incident detection is often limited by sparse sensor coverage, and reporting incidents to emergency response systems is labor-intensive. We propose to mine tweet texts to extract incident information on both highways and arterials as an efficient and cost-effective alternative to existing data sources. This paper presents a methodology to crawl, process and filter tweets that are accessible by the public for free. Tweets are acquired from Twitter using the REST API in real time. The process of adaptive data acquisition establishes a dictionary of important keywords and their combinations that can imply traffic incidents (TI). A tweet is then mapped into a high dimensional binary vector in a feature space formed by the dictionary, and classified into either TI related or not. All the TI tweets are then geocoded to determine their locations, and further classified into one of the five incident categories.
We apply the methodology in two regions, the Pittsburgh and Philadelphia Metropolitan Areas. Overall, mining tweets holds great potentials to complement existing traffic incident data in a very cheap way. A small sample of tweets acquired from the Twitter API cover most of the incidents reported in the existing data set, and additional incidents can be identified through analyzing tweets text. Twitter also provides ample additional information with a reasonable coverage on arterials. A tweet that is related to TI and geocodable accounts for approximately 5% of all the acquired tweets. Of those geocodable TI tweets, 60–70% are posted by influential users (IU), namely public Twitter accounts mostly owned by public agencies and media, while the rest is contributed by individual users. There is more incident information provided by Twitter on weekends than on weekdays. Within the same day, both individuals and IUs tend to report incidents more frequently during the day time than at night, especially during traffic peak hours. Individual tweets are more likely to report incidents near the center of a city, and the volume of information significantly decays outwards from the center.
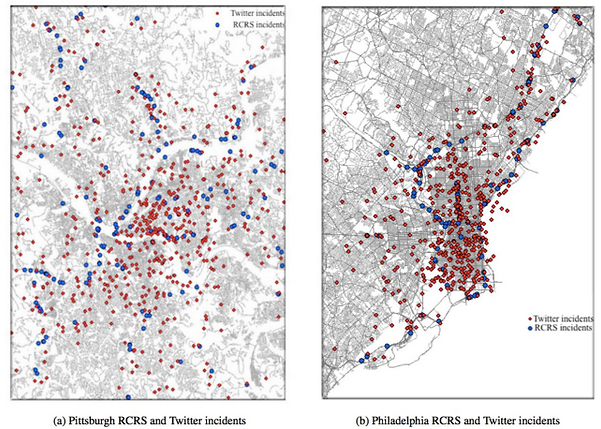
Publication
-
Yiming Gu, Sean Qian, Feng Chen (2016), “From Twitter to Detector: Real-time incident detection using social media data”, Transportation Research Part C, Vol. 67, pp.321-342. [URL]
-
Zhen (Sean) Qian (2016), “Real-time Incident Detection Using Social Media Data”, the Pennsylvania Department of Transportation (PennDOT). [URL]